Yuan-Hong Liao
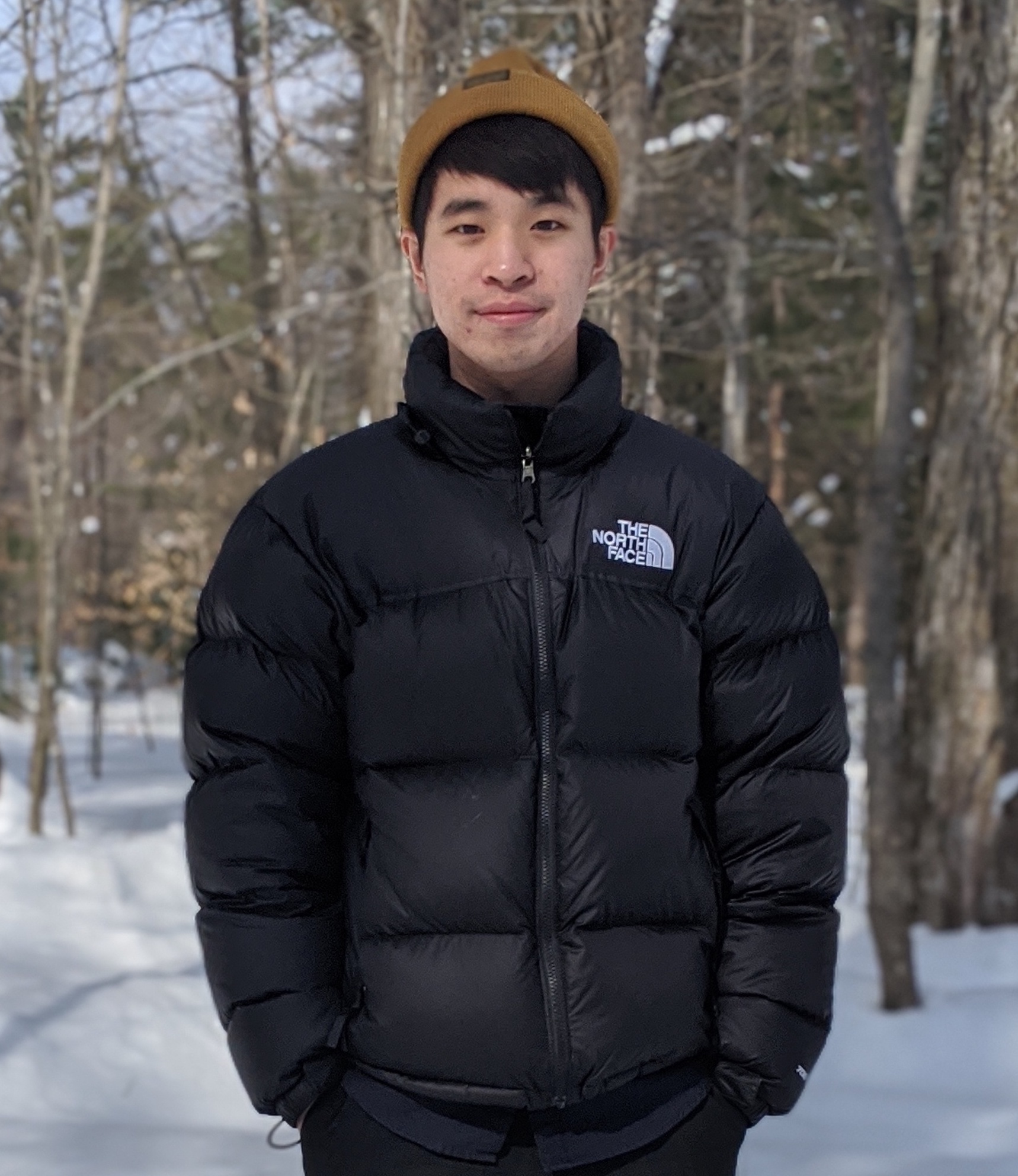
Toronto, Canada
I will be in Vienna for ICLR’24. Drop me an email if you’d like to have a chat or grad a coffee
I am a Ph.D. candidate at the University of Toronto, supervised by Prof. Sanja Fidler. I believe that the smarts of our machines are a mirror of our own thoughts, especially when we feed them with carefully curated and labeled datasets and interact with them in meaningful ways. Therefore, my reserach intersts lies in improving dataset labels both in precision and consistency and helping machine to be a better human feedback listener.
Previously, I worked at Vector Institute and USC as a visiting student in 2018 and 2017 respectively blog posts. Before my Ph.D. journey, I studied Electrical Engineering in National Tsing Hua University, supervised by Prof. Min Sun.
news
Apr 09, 2024 | ![]() |
---|---|
Jan 15, 2024 | ![]() |
Oct 01, 2023 | ![]() |
Jul 31, 2022 | ![]() |
Feb 28, 2022 | ![]() |